Shengyue Chen, Jinliang Huang, Jr-Chuan Huang
Journal of Hydrology
https://www.sciencedirect.com/science/article/pii/S0022169423006765
Published: July 2023
Abstract
There is a scarcity of streamflow data owing to the limited availability of gauge networks or delayed gauging in most parts of the world. To overcome this challenge and reproduce long-duration daily streamflow in both ungauged and poorly gauged watersheds, we proposed a novel approach that couples the process-based model Soil and Water Assessment Tool (SWAT) and the interpretable machine learning (ML) model long short-term memory (LSTM). The watershed process features generated by SWAT were combined with meteorological features as inputs for LSTM. The coupled SWAT-LSTM approach was first developed in a data-rich coastal watershed in Fujian Province, China. During the testing period, the obtained Nash-Sutcliffe efficiency coefficient (NSE) of SWAT-LSTM is 0.885, which outperformed other SWAT-MLs (e.g., backward propagation neural network, NSE = 0.843; random forest, NSE = 0.838) and calibrated SWAT (NSE = 0.706) used as comparators. Precipitation is considered the most important feature to local streamflow from a ML perspective. The pre-trained SWAT-LSTM presented satisfactory performances over 30 years of simulations in 24 hypothesized data-scarce watersheds. In ungauged watersheds, the NSE ranged from 0.474 to 0.898, with a mean of 0.685. In poorly gauged watersheds, the pre-trained SWAT-LSTM was optimized using limited local observations by introducing the transfer learning technique, and the NSE ranged from 0.591 to 0.918, with a mean of 0.760, which was markedly more accurate than the new trained models locally. Spatial proximity and physical similarity should be considered simultaneously when selecting the optimal source for data-scarce watersheds, as better performance can be achieved in less time than with tandem trained the observations of all sources. This study demonstrates that coupling SWAT with interpretable LSTM enhances the modeling confidence and provides a potential shortcut to achieving long-duration streamflow simulations in both ungauged and poorly gauged watersheds.
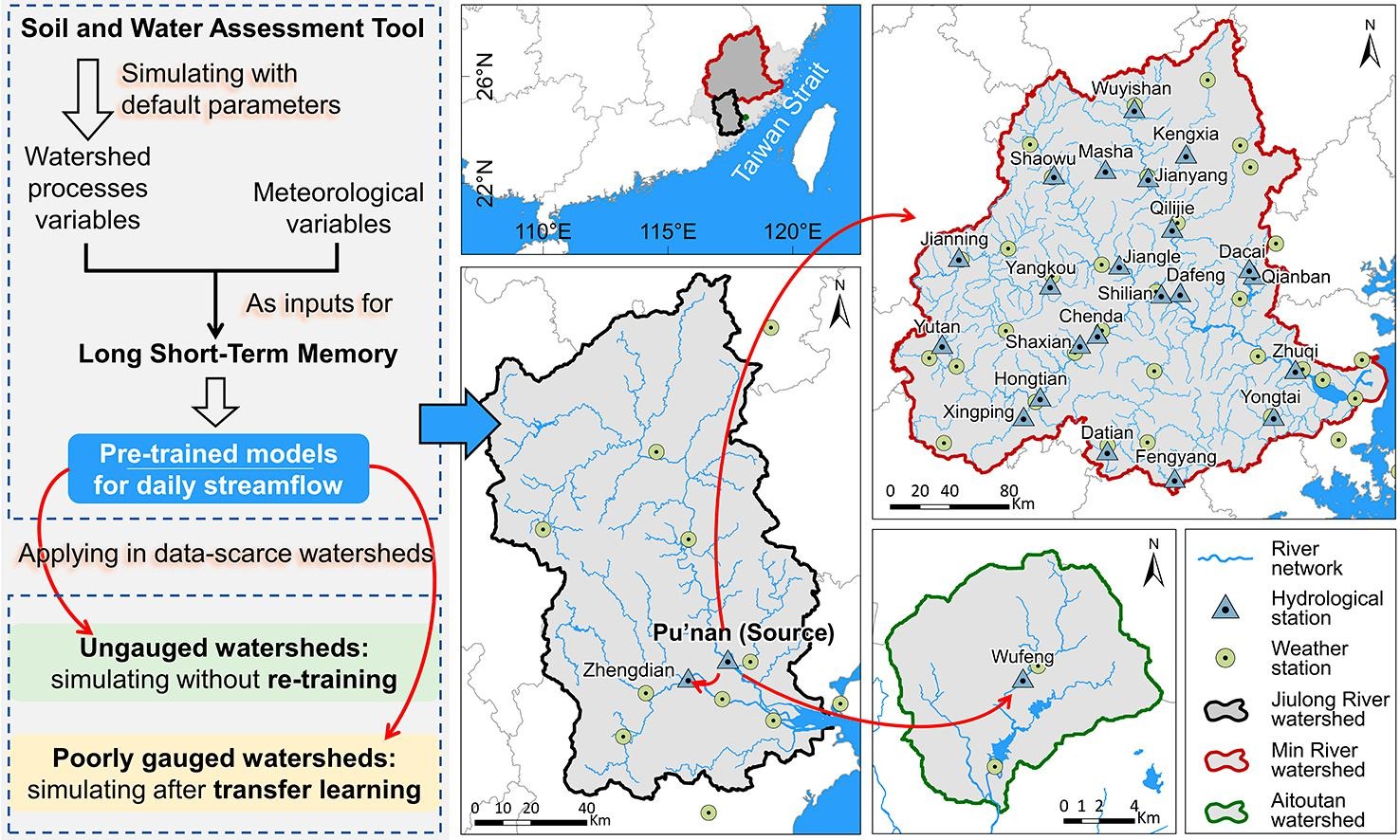